Economics is a stately subject, one that has altered little since its modern foundations were laid in Victorian times. Now it is changing radically. Standard economics is suddenly being challenged by a number of new approaches: behavioral economics, neuroeconomics, new institutional economics. One of the new approaches came to life at the Santa Fe Institute: complexity economics.
Complexity economics got its start in 1987 when a now-famous conference of scientists and economists convened by physicist Philip Anderson and economist Kenneth Arrow met to discuss the economy as an evolving complex system. That conference gave birth a year later to the Institute’s first research program – the Economy as an Evolving Complex System – and I was asked to lead this. That program in turn has gone on to lay down a new and different way to look at the economy.

To see how complexity economics works, think of the agents in the economy – consumers, firms, banks, investors – as buying and selling, producing, strategizing, and forecasting. From all this behavior markets form, prices form, trading patterns form: aggregate patterns form. Complexity economics asks how individual behaviors in a situation might react to the pattern they together create, and how that pattern would alter itself as a result, causing the agents to react anew.
This is a difficult question, so, traditionally, economics has taken up a simpler one. Conventional economics asks how agents’ behaviors (actions, strategies, forecasts) would be upheld by – would be consistent with – the aggregate patterns these cause. It asks, in other words, what patterns would call for no changes in micro-behavior, and would therefore be in stasis or equilibrium.
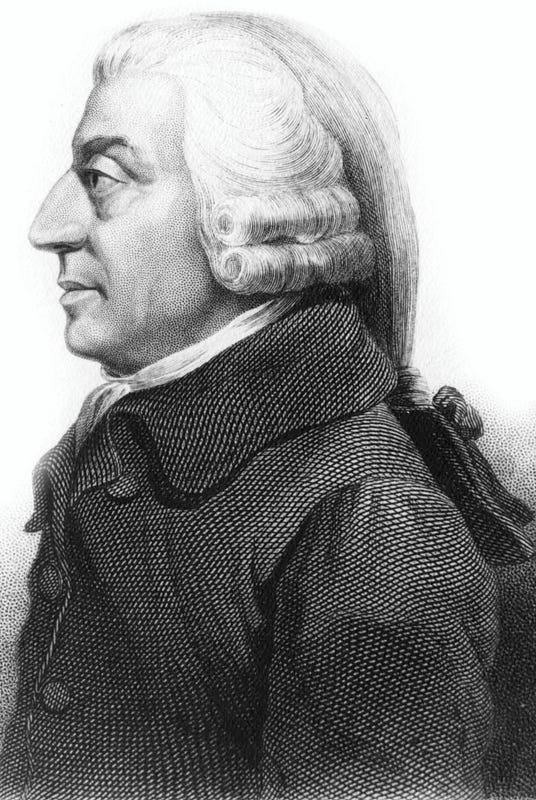
The standard, equilibrium approach has been highly successful. It sees the economy as perfect, rational, and machine-like, and many economists – I’m certainly one – admire its power and elegance. But these qualities come at a price. By its very definition, equilibrium filters out exploration, creation, transitory phenomena: anything in the economy that takes adjustment – adaptation, innovation, structural change, history itself. These must be bypassed or dropped from the theory.
Just what that change would consist of we were not quite sure when our program began. We knew we wanted to create an economics where agents could react to the outcomes they created, where the economy was always forming and evolving and not necessarily in equilibrium. But we didn’t quite know how to achieve that.
In fact, in 1988 the Institute was still very much a startup. The program consisted in its first two years of 20 or so people, several of whom proved central: John Holland, Stuart Kauffman, David Lane, and Richard Palmer. We would meet, in an early version of what became Santa Fe style, in the kitchen of the old convent on Canyon Road in the late mornings and loosely discuss ways forward.
These “emerged” slowly – sometimes painfully – mainly by talking over why economics did things the way it did and how alternatives might work. Our group was motley, even eccentric. Halfway through the first year the journalist James Gleick asked me how I would describe my group. I was hard put to reply. He pressed the question. Finally I said, “Your remember the bar in Star Wars, at the end of the galaxy with all the weird creatures, Chewbacca and the others? That’s our group.”
We did have some tools. We had new stochastic dynamic methods, and nonlinear dynamics, and novel ideas from cognitive science. And of course we had computers. But it took us a couple of years before we realized we were developing an economics based not just on different methods, but on different assumptions.
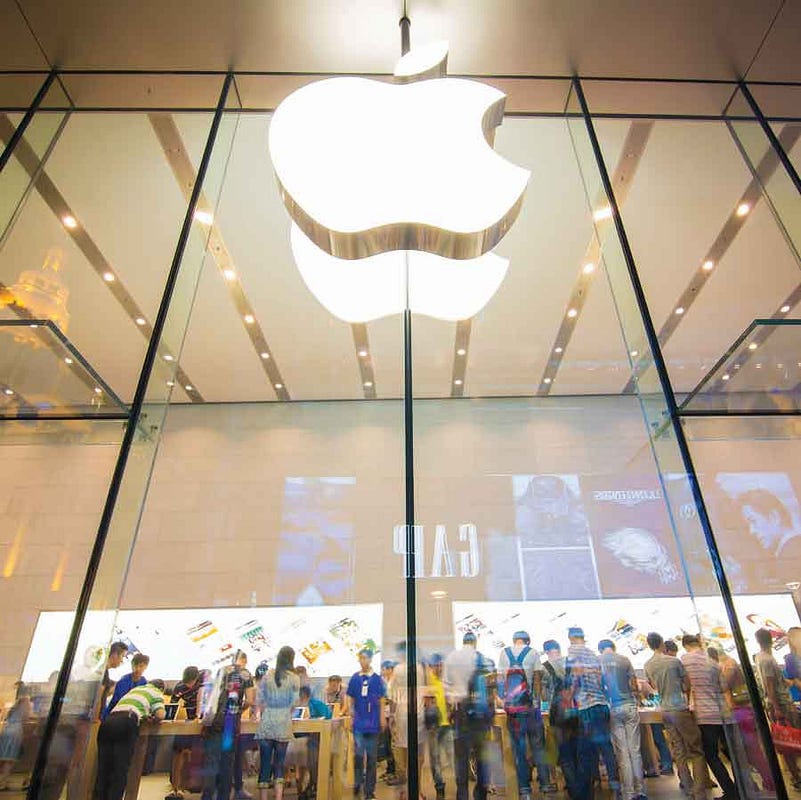
Instead of seeing agents in the economy as facing perfect, well-defined problems, we allowed that they might not know what situation they were in and would have to make sense of it. Instead of assuming agents were perfectly rational, we allowed there were limits to how smart they were. Instead of assuming the economy displayed diminishing returns (negative feedbacks), we allowed that it might also contain increasing returns (positive feedbacks). Instead of assuming the economy was a mechanistic system operating at equilibrium, we saw it as an ecology – of actions, strategies, and beliefs competing for survival – perpetually changing as new behaviors were discovered.
Other economists – in fact some of the greats like Joseph Schumpeter – had looked at some of these different assumptions before, but usually at one assumption at a time. We wanted to use all these assumptions together in a consistent way. And other complexity groups in Brussels, France, Ann Arbor, and MIT were certainly experimenting with problems in economics. But we had the advantage of an interdisciplinary critical mass for a program that ran across all of economics. The result was an approach that saw economic issues as playing out in a system that was realistic, organic, and always evolving.
Sometimes we could reduce the problems we were studying to a simple set of equations. But just as often our more challenging assumptions forced us to study them by computation. We found ourselves creating “artificial worlds” – miniature economies within the computer – where the many players would be represented by little computer programs that could explore, respond to the situation they together created, and get smarter over time.
One early computer study we did was a model of the stock market. In a stock market, investors create forecasts from the available information, make bids and offers based on these, and the stock’s price adjusts accordingly. Conventional theory assumes homogeneous investors who all use identical forecasts (so-called “rational expectations” ones) that are consistent with – on average validated by – the prices these forecasts bring about. This gives an elegant theory, but it begs the question of where the identical forecasts come from. And it rules out transitory phenomena seen in real markets, such as bubbles and crashes and periods of quiescence followed by volatility.
We decided to have “artificial investors” in our computer create their own individual forecasts. They would start with random ones, learn which worked, form new ones from these, and drop poorly performing ones. Forecasts would thus “compete” in a mutually-created ecology of forecasts. The question was how would such a market work? Would it duplicate the standard theory? Would it show anything different?
When we ran our computerized market, we did see outcomes similar to those produced by the standard theory. But we saw other phenomena, ones that appeared in real markets. Some randomly-created forecasts might predict upward price movement if previous prices were trending up; other types of forecasts might foretell a price fall if the current price became too high. So if a chance upward movement appeared, the first type would cause investors to buy in, causing a price rise and becoming self-affirming. But once the price got too high, the second sort of forecast would kick in and cause a reversal. The result was bubbles and crashes appearing randomly and lasting temporarily.
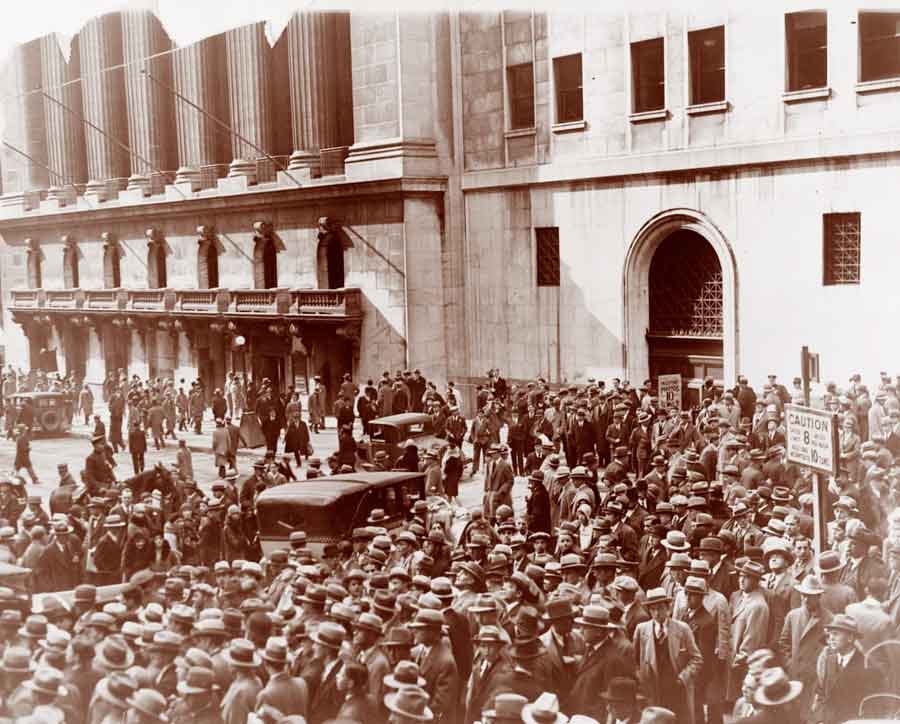
Similarly, periods of quiescence and volatility spontaneously emerged. Our investors were continually exploring for better forecasts. Most of the time this created small perturbations. But occasionally some would find forecasts that would change their behavior enough to perturb the overall price pattern, causing other investors to change their forecasts to re-adapt. Cascades of mutual adjustment would then ripple through the system. The result was periods of tranquility followed randomly by periods of spontaneously generated perturbation – quiescence and volatility.
The program, as it developed, studied many other questions: the workings of double-auction markets; the dynamics of high-tech markets; endogenously-created networks of interaction; inductive reasoning in the economy. In an SFI program parallel to ours, Josh Epstein and Rob Axtell created an artificial society called “Sugarscape” in which cooperation, norms, and other social phenomena spontaneously emerged. And in 1995 John Miller and Scott Page started an annual workshop in computational social sciences at SFI where postdocs and graduate students could get practical training in the new methods.
The approach finally received a label in 1999, when an editor at Scienceasked me on the phone to give it a name. I suggested “complexity economics,” and that name stuck.
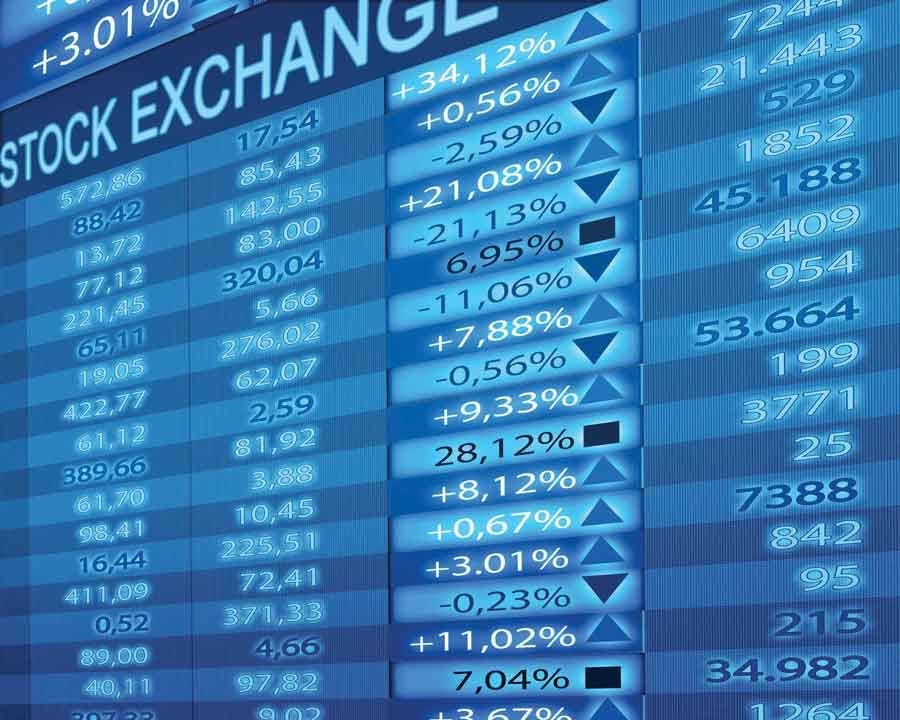
Things have widened a great deal since then. Doyne Farmer has taken up studies of how technologies improve over time. And he, Axtell, and others have been using large datasets, along with agent-based modeling methods, to understand the recent housing-market crisis. Other groups in the U.S. and Europe have been using complexity methods to look at economic development, public policy, international trade, and economic geography.
None of this means the new, nonequilibrium approach has been easily accepted into economics. The field’s mainstream has been interested but wary of it. This changed in 2009 after the financial meltdown when, as theEconomist magazine observed dryly, the financial system wasn’t the only thing that collapsed; standard economics had collapsed with it. Something different was needed, and the complexity approach suddenly looked much more relevant.
Where does complexity economics find itself now? Certainly, many commentators see it as steadily moving toward the center of economics. And there’s a recognition that it is more than a new set of methods or theories: it is a different way to see the economy. It views the economy not as machine-like, perfectly rational, and essentially static, but as organic, always exploring, and always evolving – always constructing itself.
Some people claim that this economics is a special case of equilibrium economics, but actually the reverse is true. Equilibrium economics is a special case of nonequilibrium and hence of complexity economics.
In 1996 an historian of economic thought, David Colander, captured the two different outlooks in economics in an allegory. Economists, he says, a century ago stood at the base of two mountains whose peaks were hidden in the clouds. They wanted to climb the higher peak and had to choose one of the two. They chose the mountain that was well defined and had mathematical order, only to see when they had worked their way up and finally got above the clouds that the other mountain, the one of process and organicism, was far higher. Many other economists besides our Santa Fe group have started to climb that other mountain in the last few years. There is much to discover.
W. Brian Arthur is an External Professor at the Santa Fe Institute and a Visiting Researcher at PARC in California. He has served on the Institute’s Science Board and Board of Trustees. Formerly at Stanford, he is the recipient of the inaugural Lagrange Prize in Complexity Science and the Schumpeter Prize in Economics. His book, Complexity and the Economy (Oxford University Press) appeared in 2014.
Economics/ Politics is a Balancing Act Between Choices - Modular-Finance is a Balancing Act...so is Moral Virtue.
Moral Virtue ia a mean that lies between two vices, one of excess and the other of deficiency, and it aims at hitting the mean both in feelings and actions. So it is hard to be good, for surely it is hard in each instance to find the mean just as it is hard to find the center of a circle. It is easy to get angry or to spend money - anyone can do that. But to act the right way toward the right person, in due proportion, at the right time, for the right reason, and in the right manner - that is not easy, and not everyone can do it.
(Aristotle, 384 - 322 BCE, Nichomachean Ethics)